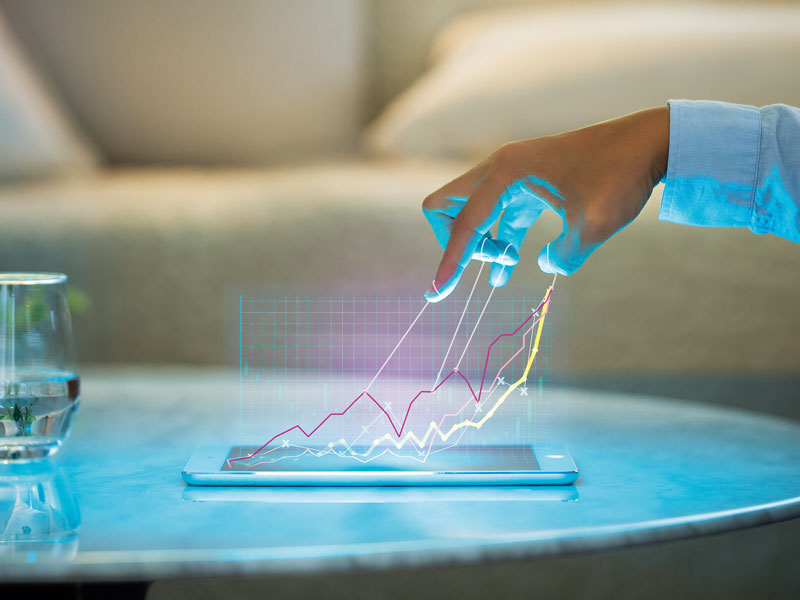
Top 5
AI and ML have the potential to modernise and transform the fund management industry, enabling, among other things, the processing and analysis of vast amounts of stock data and counteracting human bias. UOBAM, a Singapore-headquartered company with around $23bn of assets under management, saw the potential in these techniques at a relatively early stage and has built up five years of experience in this area. The decision to go down this path and to invest in the people and systems needed to make the vision a reality was a bold one, but the strategy has started to reap rewards. Notably, two of the company’s flagship funds that use AI and ML tools more than doubled returns versus their respective benchmarks in 2023. World Finance spoke with fund manager Paul Ho, Head of Investment Technology at UOB Asset Management, about the company’s development and adoption of AI and ML, its experiences so far, and how the application of these tools might be extended in future.
UOBAM began exploring AI and ML in 2019, before many in the Asian asset management industry were doing so. What thought processes were driving this move?
UOBAM has for some years shown a commitment to technology-driven customer services and business initiatives. In 2019, for example, the firm started development work on UOBAM Invest for Corporates and UOBAM Invest for Individuals. These are highly innovative robo-advisory platforms designed to offer new and easier ways to invest digitally. The platforms were subsequently launched in 2020 and 2021, respectively. Around the same time, UOBAM watched with much interest when big data, AI and ML started to be discussed to supplement human analyses. Consequently, a project was initiated within the company to test whether such tools could be used to help generate above-benchmark portfolio returns in a reliable way. The tests proved positive, and as a result we started to use them formally within our asset management process in 2020.
What were the key challenges associated with adopting an AI model, for example, in recruiting the right people?
Innovation naturally means change, so the key challenge to adopting any form of innovation is the ability to institute a shared objective of future-readiness and a culture of experimentation across the entire organisation. At UOBAM, one way this was achieved was to implement the T-shaped team structure as recommended by the CFA Institute.
This structure is based on the understanding that investment teams and technology teams can find it difficult to work together, given their different skill sets. By establishing an Invest Tech team that comprised both functions, UOBAM has been able to align all stakeholders and bridge the gap between traditional and modern approaches to investment management.
And what were the main risks that you perceived?
The risk is of course that the AI model and AI-Augmentation process does not deliver the desired results. But part of experimentation is the acceptance of failure and uncertainties. And the key to overcoming such risks is patience and rigour. After going live with our framework, we waited for three years to establish a consistent track record before marketing our AI capabilities. During that time, we closely tracked our performance and made refinements along the way.
How do funds managed by UOBAM benefit from application of the AI-ML model?
UOBAM’s AI-ML model helps to enhance our Asia Equity portfolio returns in four key ways: coverage, predictability, neutrality and scalability. There are over 25,000 stocks listed in Asia Pacific, and it is impossible for a team of analysts, however diligent, to adequately research even a small percentage of them. UOBAM uses AI-ML tools to ingest billions of data points and analyse thousands of variables related to the Asia equities universe. In this way, patterns are detected that can help predict a stock’s return potential.
The model also helps to offer an alternative to preconceived strategies such as style, geographic or technical investing. By offering an unbiased perspective, UOBAM’s model helps to prevent unfamiliar market events from being disregarded or overlooked. Finally, UOBAM’s AI-ML model can be adapted to fulfil different investment tasks. The reliance on empirical data means that it is possible to improve not just stock selection using these techniques, but also asset allocation, hedging and risk management.
How do you successfully combine and balance the input of people and machines when creating an investment portfolio?
UOBAM’s AI-Augmentation framework is different from other asset managers; it combines human expertise and AI-generated insights in a disciplined and structured way. All stock picks generated by either the manager or the machine are scored according to their ability to outperform the benchmark. Risk constraints are included to ensure that portfolio risks are managed at all times. Portfolio performance is then closely tracked to enable models to be refined as part of a human-and-machine learning process.
Two of UOBAM’s flagship funds – the United Asia Fund and the United Greater China Fund – use AI and ML tools to enhance investment performance. How have these funds performed in recent years and to what extent can their performance be attributed to AI?
AI-Augmentation was introduced into the Asia Equities fund management process in the third quarter of 2020. Both the United Asia Fund and the United Greater China Fund slightly underperformed their respective benchmarks in 2022, but strongly outperformed in 2021, 2023 and in the current year to date.
In both cases, our investigations found that the weaker 2022 performance was due to inconsistencies between the model and manager input. These inconsistencies have since been corrected, leading to stellar performances in 2023 and so far in 2024. Both funds are currently in the top quartile versus peers on a year-to-date, one-year and three-year basis, and are rated five stars by Morningstar. The United Greater China Fund won the Refinitiv Lipper award for Best Fund over Three Years in the Equity Greater China category in 2023.
Risk constraints are included to ensure that portfolio risks are managed at all times
What measures have you put in place to ensure strong governance of your AI-Augmentation framework?
At UOBAM, the Data and Digitalisation team looks at implementing group-wide AI and Data Analytics (AIDA) procedures. These are to be adopted by all AIDA project teams in the firm, including those from the investment technology teams. These procedures prescribe the necessary steps, gates and related documentation across all stages of the machine learning model lifecycle that the project team needs to adopt. This includes having an independent peer review step during the model development stage. The governance of our AI-Augmentation framework is undertaken by a region-wide Investment Technology Committee. This committee oversees the launch, development and ongoing performance of all AI-Augmentation funds.
What external factors, such as an evolving regulatory environment, do you need to be aware of when employing AI-ML tools?
As we operate in the regulated financial industry and embed AI in our products and processes, we need to be aware of developments in this fast-evolving technology and related tools. We closely monitor updates from our regulator, the Monetary Authority of Singapore, and adhere to its Fairness, Ethics, Accountability and Transparency (FEAT) principles in the responsible use of AIDA.
What potential do you see for extending the AI-Augmentation framework into other areas of investment management going forward?
We have started to apply the framework to asset allocation decision-making. The United Singapore Dynamic Income Fund (USDIF), launched late last year, uses AI techniques to allocate across multiple Singapore asset classes including real estate, fixed income and equities. Similar techniques can eventually help with regional and sector-based allocation decisions. AI-Augmentation is also being tested for applicability in our Asia Fixed Income portfolios.
UOBAM has been working with Google on advanced hedging models. What have you achieved from this collaboration to date?
Last year, we collaborated with Google on a proof of concept to investigate how more advanced algorithms could be used to improve hedging decisions. The results were encouraging, and we are planning further collaborations with Google and other technology firms to continue to build out UOBAM’s AI-Augmentation capabilities. Our AI journey is really still in its early days.